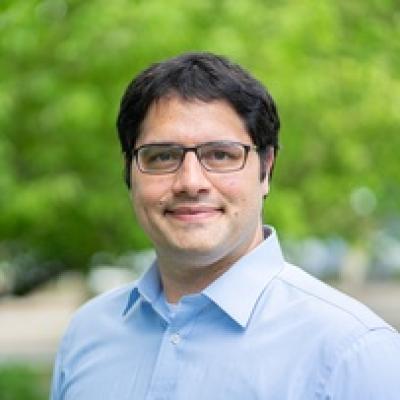
Philip Thomas
Doctoral Program Director
740 N. Pleasant Street
Amherst , MA 01003
United States
Research Areas
About
Philip Thomas's research interests are in reinforcement learning, decision-making, and AI safety. He is most interested in designing reinforcement learning algorithms that are more biologically plausible than existing algorithms, or which provide various forms of safety guarantees that make them viable for high-risk applications (e.g., medical applications). Towars these goals he has performed extensive work on (high-confidence) off-policy policy evaluation methods, with preliminary experiments for both digital marketing and medical applications. He has also studied methods for performing deep reinforcement learning without the need for the biologically implausible propagation of information backwards through the neural network.
Thomas spent 2015-2017 as a postdoctoral researcher at Carnegie Mellon University. He joined the Manning College of Information and Computer Sciences at UMass Amherst in Fall 2017 as an assistant professor.
Thomas has published in top AI conferences and journals, including the prestigious journal Science, and testified to the U.S. House Committee on Financial Services, Task Force on Artificial Intelligence in 2020. He is Co-PI on an Army Research Grant (IoBT), an NSF grant (FMitF), and the Armstrong Award. He has also received significant funding from Adobe Research. Thomas regularly serves as an area chair for NeurIPS and ICML, and served as the workshops co-chair for RLDM 2019. He has served as a reviewer for NeurIPS, ICML, AAAI, IJCAI, UAI, IROS, ICLR, RLDM, Nature, JAIR, MLJ, and JMLR.