Mass AI&Sec SP'25 Seminar: Om Thakkar, Privacy Leakage in Speech
Content
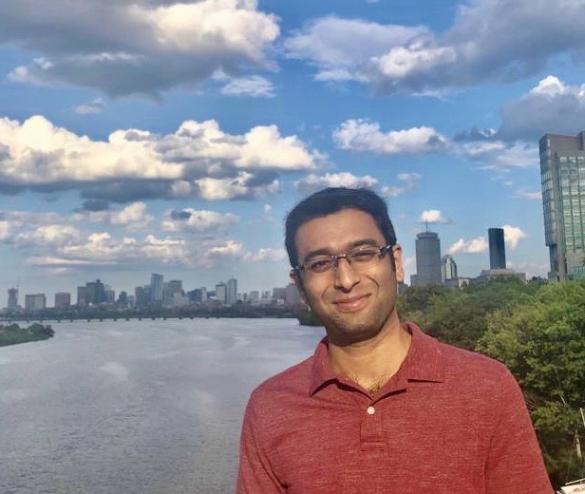
Abstract
Recent research has highlighted the vulnerability of neural networks to unintended memorization of training examples, raising significant privacy concerns. In this talk, we first explore two primary types of privacy leakage: extraction attacks and memorization audits. Specifically, we examine novel extraction attacks targeting speech models and discuss efficient methodologies for auditing memorization. In the second half of the talk, we will present empirical privacy approaches that enable training state-of-the-art speech models while effectively reducing memorization risks.
Bio
Om Thakkar is a Member of Technical Staff at OpenAI, specializing in privacy-preserving AI research with a focus on differential privacy and its applications to deep learning in production systems. Prior to joining OpenAI, he was a Senior Research Scientist at Google. He earned his Ph.D. in Computer Science from Boston University in 2019, under the guidance of Dr. Adam Smith. His doctoral research focused on differential privacy and its applications to machine learning. Dr. Thakkar holds a B.Tech. in Information and Communication Technology from the Dhirubhai Ambani Institute in India, completed in 2014. He has published his research in leading conferences such as the IEEE Symposium on Security and Privacy (S&P), NeurIPS, and ICML and has several patents under his name.