Data-driven Approaches for Energy-aware Application Management in Cloud and Edge Systems
Content
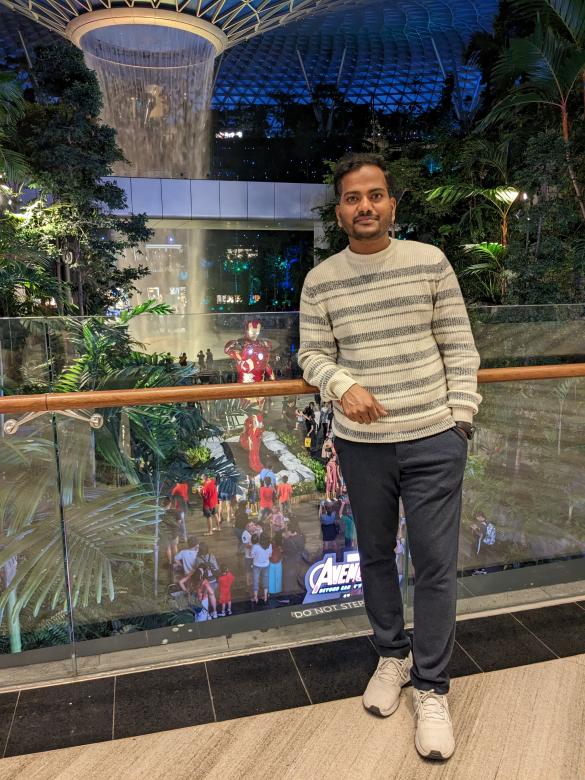
Speaker
Abstract
Contemporary large-scale computing systems are becoming increasingly heterogeneous and decentralized, driven by the growing demand for digitization, particularly for low-latency smart applications.. This trend is exemplified by the deployment of edge micro Data Centers (DCs) alongside existing hyper-scale cloud DCs. These cyber-physical infrastructures consume substantial energy and significantly contribute to carbon emissions. Assessing and managing energy use in such large, distributed systems presents numerous challenges including, hyper-heterogeneity of hardware and power characteristics, diversity of user workloads and software stacks, and potential performance degradation from energy-saving measures. Traditional energy-efficient resource management solutions, based on human-designed heuristic algorithms or rule-based policies, prove inadequate for dynamic, large-scale complex systems. However, the advent of machine learning (ML) and the availability of extensive monitoring data have created opportunities for data-driven, ML-centric resource management techniques. In this talk, I will explore our recent research in developing data-driven models and optimization strategies for efficient energy management in large-scale cloud and edge computing systems. I will discuss how these approaches can address the challenges of heterogeneity, and performance optimization while significantly reducing energy consumption and carbon footprint in modern computing infrastructures.
Bio
Dr. Shashikant Ilager is a postdoctoral researcher in HPC Research group at TU Wien, Austria. Previously, he completed his PhD with a focus on ML-based energy and thermal efficient cloud data center resource management at the CLOUDS Lab, University of Melbourne, Australia, in 2021. His research interests span the intersection of large-scale distributed systems and machine learning, where he investigates various resource management techniques focusing on sustainability. As a systems researcher, his goal is to design and implement automated, data-driven resource management algorithms and systems. His current research emphasizes green and cost-effective computational methods across the cloud-edge continuum. His work, grounded on characterizing distributed systems and his research methods, encompasses the computing stack—from individual nodes to large-scale data centers—and extends to the development of hardware-software co-design methods for executing application workloads fast and resource efficiently.