UMass AI&Sec SP'25 Seminar: Anshuman Suri, White-box v/s Black-box: Privacy Auditing for Machine Learning
Content
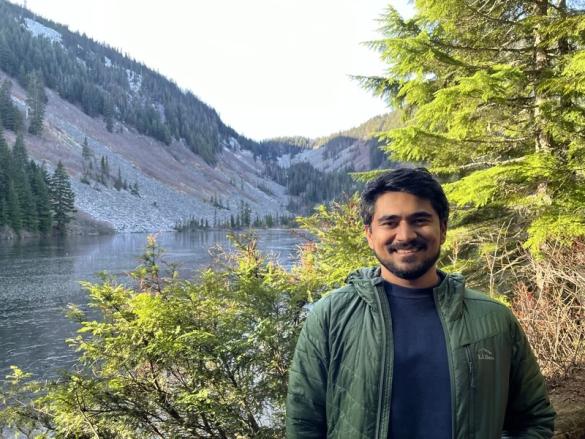
Speaker
Title
White-box v/s Black-box: Privacy Auditing for Machine Learning
Abstract
Machine learning models pose privacy risks through memorization, with membership inference being the most studied threat—determining whether a specific record was in the training data. State-of-the-art attacks assume black-box access, and prior theoretical work suggests that parameter access is unnecessary for optimal membership inference. This view is reinforced by prevailing research folklore, with little work exploring MIAs under parameter access. In this talk, I will challenge these assumptions and demonstrate that, contrary to common belief, optimal membership inference does require parameter access. I will then discuss the implications for privacy auditing and how it differs from inference attacks designed for adversarial or
demonstrative purposes.
Bio
Anshuman Suri is a postdoctoral fellow at Northeastern University’s Khoury College of Computer Sciences, working with Alina Oprea. He earned his PhD from the University of Virginia in 2024 under David Evans, focusing on security and privacy in machine learning. His research spans membership inference, user inference, and attacks on large language models. He has worked as an Applied Scientist at Microsoft and interned at Oracle Research. His work has received several honors, including the John A. Stankovic Graduate Research Award. He has also served as a reviewer for top ML conferences, earning Outstanding Reviewer awards at ICLR, ICML, and ICCV.
Host