Sheldon/UMass COVID-19 Forecasting Model Ranked #1 in PNAS Study
Content
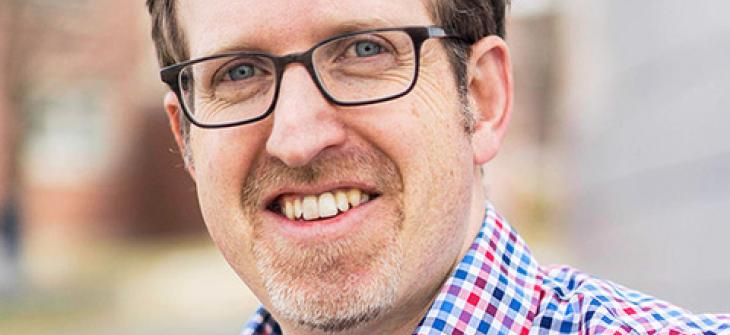
A COVID-19 forecasting model developed by Associate Professor Daniel Sheldon of the Manning College of Information and Computer Sciences (CICS) was found to be the most accurate individual model for forecasting incident deaths from April 2020 through October 2021, according to a recent study published in Proceedings of the National Academy of Sciences (PNAS).
In March 2020, when only a few models existed to inform health authorities and the general public about the development of the COVID-19 pandemic, Sheldon realized that he had “the desire and the skills” to build models to help answer questions about what might happen next. He reached out to Nicholas Reich, an associate professor from the UMass Amherst School of Public Health and Health Sciences, and began work with Reich and Graham Gibson, then a doctoral student at the ReichLab. Together, they developed a novel prediction model to forecast real-time trends in COVID-19 deaths, the UMass MechBayes (mechanistic Bayesian) model.
MechBayes, which began producing forecasts in late April of 2020, quickly became one of the most accurate individual models according to the COVID-19 ForecastHub — a joint project of Reich’s laboratory at UMass Amherst and the U.S. Centers for Disease Control (CDC) — and received funding from the NIH. It has contributed to ensemble forecasts used by the CDC and was featured on FiveThirtyEight.com. In the PNAS study, MechBayes’ performance was the highest of all individual models and very close in accuracy to the ensemble. Sheldon called this a “huge win for the modern probabilistic programming and Bayesian inference tools used to build MechBayes,” noting that the underlying epidemiological model was similar to a number of other teams.
Their new model built upon SEIR, a classical “compartmental” mechanistic model for diseases, which assumes that every individual is in one of a mutually exclusive set of compartments (Susceptible, Exposed, Infected, or Recovered), and focuses on the rates of flow between compartments. The team added two additional compartments, to model deaths and to more accurately account for the time delay between infection and death.
With their new model, they worked to overcome two challenges of forecasting during the COVID-19 pandemic. First, large changes in transmission occurred throughout the pandemic due to public health interventions, changes in human behavior, vaccinations, and changes in the virus itself. Second, the relationship between reported cases and deaths also changed over time due to changes in test availability and reporting behavior, new treatments, vaccinations, and many other factors. In order to tailor the model to these challenges, as well as the lack of historical information on COVID-19, they designed MechBayes within a Bayesian framework to respond to real-time evidence about cases and deaths. As a Bayesian model—which continually updates its beliefs based on new information—MechBayes was able to respond to changes in interventions and behavior, and account for uncertainty in its beliefs.
After submitting forecasts weekly for nearly two years to the COVID-19 ForecastHub, the team decided to retire the highly successful model in March 2022, owing to the diminishing importance of forecasts to public health decision-making.
“I’m proud that as a computer scientist, I could play a supporting role in this effort to produce forecasts that were used by the CDC,” says Sheldon, noting that he spent hundreds of hours developing the model and generating weekly forecasts. “Working with Nick Reich and his lab, I learned a lot about the care, testing, and validation needed to make predictions that are used by people and could impact public health. Nick is a leader in his community and really emphasizes validation, falsifiability, and social impacts of predictions — providing a great lesson for machine learning practitioners who make predictions used by people.”
Sheldon joined CICS as faculty in 2012, and received an NSF CAREER award in 2018. He has received national attention for his work using artificial intelligence and public weather data to track bird migration patterns. Previously, he was a postdoctoral fellow in the School of Electrical Engineering and Computer Science at Oregon State University, where he held a National Science Foundation fellowship in bioinformatics. He received his doctorate in computer science from Cornell University in 2009, and his bachelor’s in mathematics from Dartmouth College in 1999.