CIIR Talk Series: Alane Suhr, Interactive Language Agents: Training, Evaluation, and Interface
Content
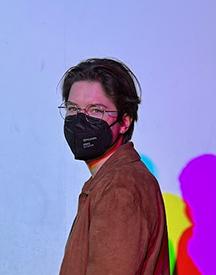
Speaker
Alane Suhr, UC Berkeley
Abstract
The increasing capability of LLMs makes them appealing for adoption in labor-intensive human tasks. For example, significant efforts have recently focused on developing agents -- systems that map observations and instructions to executable actions -- and their benchmarks in real-world tasks like web navigation. In this talk, I will discuss recent work in training and improving such models through interactions with human users, and developing better evaluations for these agents, which in turn can be used to automatically improve agent performance without requiring any demonstration data or human annotation. However, in developing systems like this, and in applying LLMs and other large pre-trained models to real-world problems, we should be aware of their fundamental limitations; for example, their sensitivity to design considerations like prompt formatting. I will detail recent work where we find that LLMs can be incredibly sensitive to arbitrary design decisions, like choices of separators or multiple choice labels.
Bio
Alane Suhr recently joined EECS and BAIR at UC Berkeley as an Assistant Professor. Alane's work focuses on building language-using systems that communicate with and learn from human users in collaborative, situated interactions. Prior to joining Berkeley, Alane completed a PhD in Computer Science at Cornell University / Cornell Tech and spent a year afterwards as a Young Investigator at the Allen Institute for AI.
Subscribe to mailing list by sending an email to ciir-talks-request [at] cs [dot] umass [dot] edu (ciir-talks-request[at]cs[dot]umass[dot]edu) with "subscribe" as the email subject (without the quotation marks) for Zoom link/passcode notifications, or click here for Zoom link and reach out to zamani [at] cs [dot] umass [dot] edu (subject: CIIR%20Talks%20Passcode) (Hamed Zamani) for the passcode.