UMass Amherst Hosts Inaugural Reinforcement Learning Conference
Content

In early August, 550 researchers gathered at the University of Massachusetts Amherst for the inaugural Reinforcement Learning Conference (RLC). Designed as a more focused setting for the sharing of research than large AI conferences, the event aimed to shine a spotlight on established and emerging topics in reinforcement learning, one of three main branches of modern AI.
"We wanted a smaller, more focused conversation instead of one of these massive AI events that have over 15,000 attendees," says Scott Niekum, associate professor at the Manning College of Information and Computer Sciences at UMass Amherst (CICS) and one of the RLC organizers. "Reinforcement learning is crucial to areas of inquiry like recommendation engines and robotics, and it needs a space where practitioners can connect with the research they care about—and each other."
When organizers Amy Zhang of the University of Texas Austin, Eugene Vinitsky of NYU, and Glenn Berseth of Université de Montréal first approached Niekum and fellow CICS faculty Phil Thomas and Bruno Castro da Silva with the idea of holding a conference on reinforcement learning at UMass Amherst, it just made sense.
"UMass is the birthplace of a modern computational approach to reinforcement learning, thanks in large part to Andrew Barto," explains Niekum, referring to the CICS professor emeritus, widely viewed as having created the field at UMass Amherst with his doctoral student Rich Sutton. Together, the pair authored the foundational 1998 textbook Reinforcement Learning: An Introduction. This now-standard textbook established reinforcement learning, where learning happens through trial and error engagement with an environment, as a formal discipline within the field of artificial intelligence. A much expanded second edition was published in 2018.
Telling the History of Reinforcement Learning
At the conference, Barto gave a keynote address providing an overview of the foundation and early growth of reinforcement learning and his own place in the story.
As he told it, Barto first encountered the idea of a computational model of a neuron, first proposed by Warren McCulloch and Walter Pitts in 1943 as an undergraduate math major in the late 1960s at the University of Michigan. This idea would change his life. "That was tremendously exciting to me, this connection between the brain, biology, and math," said Barto. "It was like, wow, I want to get involved in this."
Barto stayed on at the University of Michigan for graduate work, joining the interdepartmental Logic of Computers Group, which investigated biologically inspired computational methods, such as genetic algorithms and neural networks. He received his doctorate in 1975 with a thesis on the computational architecture known as cellular automata. After hearing of a postdoctoral opportunity at UMass Amherst, he moved to Western Massachusetts to join a team of computer science professors under contract with the Air Force Wright Avionics Laboratories to explore a "crazy idea" from a senior scientist at the Air Force—that neurons might be understood as "hedonists" that "work to maximize some analog of pleasure while minimizing some analog of pain."
The end result, co-authored with Sutton and nicknamed "the yellow report" by the Air Force, pointed the way towards not only the creation of algorithms based on reinforcement learning but significant collaborations with the fields of psychology and neuroscience. Barto and Sutton authored a breakthrough 1981 paper in Psychological Review, "Toward a Modern Theory of Adaptive Networks: Expectation and Prediction," after consulting with John Moore, a professor of psychological and brain sciences at UMass Amherst and expert in animal learning. This paper led to the creation of temporal difference (TD) learning, which not only contributed to the field of artificial intelligence but became the foundation in neuroscience for understanding how dopamine signals function in the brain.
Despite these contributions to multiple fields, Barto is known for his humility. As his longtime collaborator and co-author Rich Sutton said at a 2019 lifetime achievement award in neuroscience presentation at UMass Amherst, "A field's success is due to more than just its research output … I've gained a [deep] appreciation for Andy's contribution in setting the tone of the field. That tone is an emphasis on scholarship, humility, and openness, on welcoming all fields and all people for whatever contributions they can make."
"I've had such wonderful students," says Barto. Aside from Sutton and numerous other leading researchers in reinforcement learning, he served as the doctoral advisor to three current CICS faculty in the late 2000s: Niekum, who now directs the Safe, Confident, and Aligned Learning + Robotics Lab and is a core member of the interdepartmental UMass Robotics Group; Thomas, the college's doctoral program director and co-director of the Autonomous Learning Lab (ALL); and da Silva, the other ALL co-director.
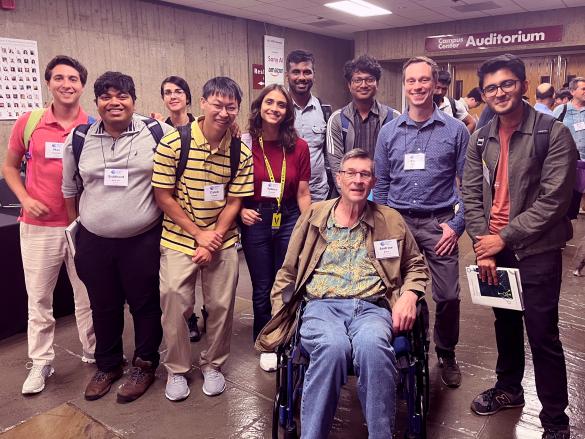
Pointing to the "Next Breakthrough" at RLC 2024
"It was exciting to see Andy give this talk and hear such a rich history of the creation of the field," says Niekum. "And I was just as excited that at this conference, we were seeing new research that honors that legacy in rethinking basic scientific tenets and questioning basic assumptions in a way that might just jostle the field into its next breakthrough."
Aside from Barto, keynote speakers included David Silver from Google Deep Mind, known for his work on the AlphaGo project, Peter Stone, a professor at the University of Texas Austin and chief scientist at Sony AI, and Emma Brunskill of Stanford University, known for her contributions to the use of reinforcement learning in healthcare and education.
Niekum praises the enthusiasm from the reinforcement learning field, calling this first conference a "huge success," with nearly 300 paper submissions, 115 accepted papers, and very active discussions—along with support from a robust list of sponsors, including Amazon, Sony AI, Google DeepMind and Google Research, Electric Sheep Robotics, Boston Dynamics, and Valence Labs. "We heard from a lot of people that it was their favorite academic conference they've ever been to," says Niekum. "It has been incredible to see how far the field has come, both in terms of the capabilities of reinforcement learning and the size of the community."
Papers from the Reinforcement Learning Conference can be found in the Reinforcement Learning Journal. The next conference will be held in 2025 at the University of Alberta, where Richard Sutton is a professor and director of the Reinforcement Learning and Artificial Intelligence Lab.