Who (or What) Searches the Search Engines of the Future?
Award-winning team introduces a cost-effective method to redesign search engines for Artificial Intelligence.
Content
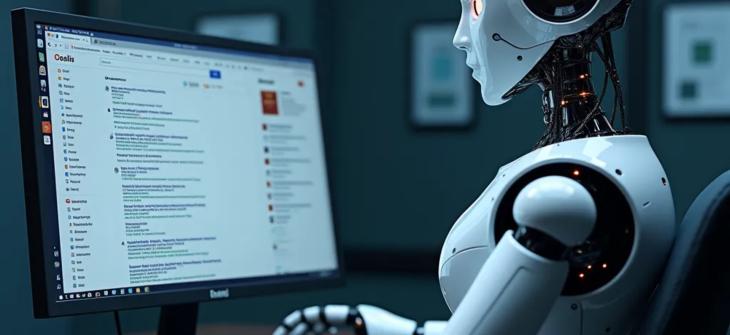
The internet search engine of the future will be powered by artificial intelligence. One can already choose from a host of AI-powered or AI-enhanced search engines—though their reliability often still leaves much to be desired. However, an award-winning team of computer scientists at the University of Massachusetts Amherst recently published and released a novel system for evaluating the reliability of AI-generated searches. Called “eRAG,” the method is a way of putting the AI and search engine in conversation with each other, then evaluating the quality of search engines for AI use.
“All of the search engines that we’ve always used were designed for humans,” says Alireza Salemi, a graduate student in the Manning College of Information and Computer Sciences at UMass Amherst and the paper’s lead author. “They work pretty well when the user is a human, but the search engine of the future’s main user will be one of the AI Large Language Models (LLMs), like ChatGPT. This means that we need to completely redesign the way that search engines work, and my research explores how LLMs and search engines can learn from each other.”
The basic problem that Salemi and the senior author of the research Hamed Zamani, associate professor of information and computer sciences at UMass Amherst, confront is that humans and LLMs have very different informational needs and consumption behavior. For instance, if you can’t quite remember the title and author of that new book that just published, you can enter a series of general search terms, such as, “what is the new spy novel with an environmental twist by that famous writer,” and then narrow the results down, or run another search as you remember more information (the author is a woman who wrote the novel “Flamethrowers”), until you find the correct result (“Creation Lake” by Rachel Kushner — which Google returned as the third hit after following the process above).
But that’s how humans work, not LLMs. They are trained on specific, enormous sets of data, and anything that is not in that data set — like the new book that just hit the stands — is effectively invisible to the LLM. Furthermore, they’re not particularly reliable with hazy requests, because the LLM needs to be able to ask the engine for more information; but to do so, it needs to know the correct additional information to ask.
This story was originally published by the UMass Amherst Office of News & Media Relations.